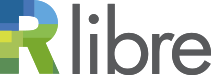
Répertoire de publications
de recherche en accès libre
de recherche en accès libre
Chachoui, Yasmine; Azizi, Nabiha; Hotte, Richard et Benseba, Tahar (2024). Enhancing algorithmic assessment in education: Equi-fused-data-based SMOTE for balanced learning.. Computer and Education : Artificial intelligence, 6, 1-15. https://doi.org/10.1016/j.caeai.2024.100222
Fichier(s) associé(s) à ce document :![]() |
PDF
- Algorithmic assessment in education.pdf
Contenu du fichier : Version de l'éditeur |
|
Catégorie de document : | Articles de revues |
---|---|
Évaluation par un comité de lecture : | Oui |
Étape de publication : | Publié |
Résumé : | Recently, there has been a growing interest among researchers in enhancing the efficacy of learning through the utilization of diverse machine learning models within the field of artificial intelligence. However, imbalanced data distributions in educational datasets present a significant challenge to machine learning algorithms. This imbalance can result in biased models, untrustworthy outcomes, and poor performance. Data was gathered from a sample of 2176 first-year novice programming students in this study. Due to an alarming 76% failure rate, the imbalanced dataset was preprocessed before being oversampled with techniques such as SMOTE, SMOTE Borderline, SMOTE-ENN, and ADASYN. The proposed non-redundant synthetic data cooperation approach, named Equi-Fused-Data-based SMOTE, seeks to capitalize on the diversity of the obtained data by combining oversampled datasets. The balanced bagging model was then applied to the combined dataset to demonstrate the robustness of this approach. The promising results demonstrate the effectiveness of the Equi-Fused-Data-based SMOTE model, which achieved a higher Accuracy of 93.85%, a Precision, Recall and F1-score of 92,86%, and an AUC of 98.08%. |
Adresse de la version officielle : | https://doi.org/10.1016/j.caeai.2024.100222 |
Déposant: | Hotte, Richard |
Responsable : | Richard Hotte |
Dépôt : | 18 avr. 2024 17:04 |
Dernière modification : | 18 avr. 2024 17:04 |
![]() |
RÉVISER |