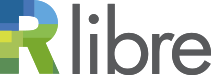
Répertoire de publications
de recherche en accès libre
de recherche en accès libre
Jemal, Imene; Abou-Abbas, Lina; Henni, Khadidja; Mitiche, Amar et Mezghani, Neila (2024). Domain adaptation for EEG-based, cross-subject epileptic seizure prediction. Frontiers in Neuroinformatics, 18, 1303380. https://doi.org/10.3389/fninf.2024.1303380
Fichier(s) associé(s) à ce document :![]() |
PDF
- Jemal2024.pdf
Contenu du fichier : Version de l'éditeur Licence : Creative Commons CC BY. |
|
Catégorie de document : | Articles de revues |
---|---|
Évaluation par un comité de lecture : | Oui |
Étape de publication : | Publié |
Résumé : | The ability to predict the occurrence of an epileptic seizure is a safeguard against patient injury and health complications. However, a major challenge in seizure prediction arises from the significant variability observed in patient data. Common patient-specific approaches, which apply to each patient independently, often perform poorly for other patients due to the data variability. The aim of this study is to propose deep learning models which can handle this variability and generalize across various patients. This study addresses this challenge by introducing a novel cross-subject and multi-subject prediction models. Multiple-subject modeling broadens the scope of patient-specific modeling to account for the data from a dedicated ensemble of patients, thereby providing some useful, though relatively modest, level of generalization. The basic neural network architecture of this model is then adapted to cross-subject prediction, thereby providing a broader, more realistic, context of application. For accrued performance, and generalization ability, cross-subject modeling is enhanced by domain adaptation. Experimental evaluation using the publicly available CHB-MIT and SIENA data datasets shows that our multiple-subject model achieved better performance compared to existing works. However, the cross-subject faces challenges when applied to different patients. Finally, through investigating three domain adaptation methods, the model accuracy has been notably improved by 10.30% and 7.4% for the CHB-MIT and SIENA datasets, respectively. |
Adresse de la version officielle : | https://www.frontiersin.org/articles/10.3389/fninf... |
Déposant: | Ayena, Johannes |
Responsable : | Johannes Ayena |
Dépôt : | 12 mars 2024 20:25 |
Dernière modification : | 12 mars 2024 20:25 |
![]() |
RÉVISER |