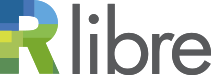
Répertoire de publications
de recherche en accès libre
de recherche en accès libre
Abou-Abbas, Lina; Henni, Khadidja; Jemal, Imene; Mitiche, Amar et Mezghani, Neila (2023). Patient-independent epileptic seizure detection by stable feature selection. Expert Systems with Applications, 232, 120585. https://doi.org/10.1016/j.eswa.2023.120585
Fichier(s) associé(s) à ce document :![]() |
PDF
- AbouAbbas_2023.pdf
Contenu du fichier : Version de l'éditeur Licence : Creative Commons CC BY-NC-ND. |
|
Catégorie de document : | Articles de revues |
---|---|
Évaluation par un comité de lecture : | Oui |
Étape de publication : | Publié |
Résumé : | The detection of epileptic seizures in EEG signals is a challenging task because it requires careful review of multi-channel EEG recordings over a lengthy time interval. In general, EEG-based seizure detection is strongly dependent on the ability to select descriptive features that are also stable in the sense that they are not sensitive to changes in the training data. This study proposes and investigates a patient-independent seizure detection model that uses stable EEG-based features obtained by comparing multiple feature selection methods. The schemes considered can be divided into five categories, often referred to as similarity, information theoretic, sparse learning, statistical, and graph centrality feature selection methods. The stability of the features these schemes produce was evaluated by random forest classification, using the intersection, frequency, correlation, and similarity measures. In experiments with Temple University Hospital’s Seizure database of EEG records of 341 patients, unsupervised graph centrality feature selection was the most effective method, with a correct classification rate of 91.5%. |
Adresse de la version officielle : | https://www.sciencedirect.com/science/article/pii/... |
Déposant: | Ayena, Johannes |
Responsable : | Neila Mezghani |
Dépôt : | 28 nov. 2023 15:53 |
Dernière modification : | 07 août 2024 13:30 |
![]() |
RÉVISER |