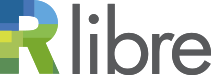
Répertoire de publications
de recherche en accès libre
de recherche en accès libre
Henni, Khadidja; Mezghani, Neila et Mitiche, Amar (2020). Cluster density properties define a graph for effective pattern feature selection. IEEE Access, 8, 62841-62854. https://doi.org/10.1109/ACCESS.2020.2981265
Fichier(s) associé(s) à ce document :![]() |
PDF
- Henni2020.pdf
Contenu du fichier : Version de l'éditeur Licence : Creative Commons CC BY. |
|
Catégorie de document : | Articles de revues |
---|---|
Évaluation par un comité de lecture : | Oui |
Étape de publication : | Publié |
Résumé : | Feature selection is a challenging problem that occurs in the high-dimensional data analysis of many major applications. It addresses the curse of dimensionality by determining a small set of features to represent high-dimensional data without significant or noticeable loss of information. The purpose of this study is to develop and investigate a new unsupervised feature selection method which uses the k-influence space concept and subspace learning to map features onto a weighted graph and rank them by importance according to the PageRank graph centrality measure. The graph design in this method promotes feature relevance, downgrades redundancy, and is robust to outliers and cluster imbalances. In K-Means classification experiments using the ASU feature selection testing datasets, the method produces better accuracy and normalized mutual information results than state-of-the-art unsupervised feature selection algorithms. In a further evaluation, using a dataset of over 14,000 tweets, conventional classification of features selected by the method gave better sentiment analysis results than deep learning feature selection and classification. |
Adresse de la version officielle : | https://ieeexplore.ieee.org/abstract/document/9037... |
Déposant: | Ayena, Johannes |
Responsable : | Johannes Ayena |
Dépôt : | 12 janv. 2023 21:19 |
Dernière modification : | 12 janv. 2023 21:19 |
![]() |
RÉVISER |