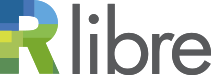
Répertoire de publications
de recherche en accès libre
de recherche en accès libre
Abou-Abbas, Lina; Henni, Khadidja; Jemal, Imene et Mezghani, Neila (2024). Generative AI with WGAN-GP for boosting seizure detection accuracy. Frontiers in Artificial Intelligence, 7, 1437315. https://doi.org/10.3389/frai.2024.1437315
Fichier(s) associé(s) à ce document :![]() |
PDF
- AbouAbbas_2024_2.pdf
Contenu du fichier : Version de l'éditeur Licence : Creative Commons CC BY. |
|
Catégorie de document : | Articles de revues |
---|---|
Évaluation par un comité de lecture : | Oui |
Étape de publication : | Publié |
Résumé : | Background: Imbalanced datasets pose challenges for developing accurate seizure detection systems based on electroencephalogram (EEG) data. Generative AI techniques may help augment minority class data to facilitate automatic epileptic seizure detection. New method: This study investigates the impact of various data augmentation (DA) approaches, including Wasserstein Generative Adversarial Network with Gradient Penalty (WGAN-GP), Vanilla GAN, Conditional GAN (CGAN), and Cramer GAN, on classification performance with Random Forest models. The best-performing GAN variant, WGAN-GP, was then integrated with a bidirectional Long Short-Term Memory (LSTM) architecture and compared against traditional and synthetic oversampling methods. Results: The evaluation of different GAN variants for data augmentation with Random Forest classifiers identified WGAN-GP as the most effective approach. The integration of WGAN-GP with bidirectional LSTM yielded substantial performance improvements, outperforming traditional oversampling methods and achieving an accuracy of 91.73% on the augmented data, compared to 86% accuracy on real data without augmentation. Comparison with existing methods: The proposed generative AI approach combining WGAN-GP and recurrent neural network models outperforms comparative synthetic oversampling methods on metrics relevant for reliable seizure detection from imbalanced EEG datasets. Conclusions: Incorporating the WGAN-GP generative AI technique for data augmentation and integrating it with bidirectional LSTM elevates seizure detection accuracy for imbalanced EEG datasets, surpassing the performance of traditional oversampling and class weight adjustment methods. This approach shows promise for improving epilepsy monitoring and management through enhanced automated detection system effectiveness. |
Adresse de la version officielle : | https://www.frontiersin.org/journals/artificial-in... |
Déposant: | Ayena, Johannes |
Responsable : | Neila Mezghani |
Dépôt : | 04 oct. 2024 14:26 |
Dernière modification : | 04 oct. 2024 14:26 |
![]() |
RÉVISER |