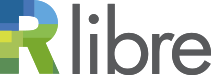
Répertoire de publications
de recherche en accès libre
de recherche en accès libre
Ben Nasr, Mohamed Chiheb; Ben Jebara, Sofia; Otis, Samuel; Abdulrazak, Bessam et Mezghani, Neila (2021). A spectral-based approach for BCG signal content classification. Sensors, 21 (3), 1020. https://doi.org/10.3390/s21031020
Fichier(s) associé(s) à ce document :![]() |
PDF
- ChihebBenNasr2021.pdf
Contenu du fichier : Version de l'éditeur Licence : Creative Commons CC BY. |
|
Catégorie de document : | Articles de revues |
---|---|
Évaluation par un comité de lecture : | Oui |
Étape de publication : | Publié |
Résumé : | This paper has two objectives: the first is to generate two binary flags to indicate useful frames permitting the measurement of cardiac and respiratory rates from Ballistocardiogram (BCG) signals—in fact, human body activities during measurements can disturb the BCG signal content, leading to difficulties in vital sign measurement; the second objective is to achieve refined BCG signal segmentation according to these activities. The proposed framework makes use of two approaches: an unsupervised classification based on the Gaussian Mixture Model (GMM) and a supervised classification based on K-Nearest Neighbors (KNN). Both of these approaches consider two spectral features, namely the Spectral Flatness Measure (SFM) and Spectral Centroid (SC), determined during the feature extraction step. Unsupervised classification is used to explore the content of the BCG signals, justifying the existence of different classes and permitting the definition of useful hyper-parameters for effective segmentation. In contrast, the considered supervised classification approach aims to determine if the BCG signal content allows the measurement of the heart rate (HR) and the respiratory rate (RR) or not. Furthermore, two levels of supervised classification are used to classify human-body activities into many realistic classes from the BCG signal (e.g., coughing, holding breath, air expiration, movement, et al.). The first one considers frame-by-frame classification, while the second one, aiming to boost the segmentation performance, transforms the frame-by-frame SFM and SC features into temporal series which track the temporal variation of the measures of the BCG signal. The proposed approach constitutes a novelty in this field and represents a powerful method to segment BCG signals according to human body activities, resulting in an accuracy of 94.6%. |
Adresse de la version officielle : | https://www.mdpi.com/1424-8220/21/3/1020 |
Déposant: | Ayena, Johannes |
Responsable : | Neila Mezghani |
Dépôt : | 12 janv. 2023 21:29 |
Dernière modification : | 07 août 2024 19:21 |
![]() |
RÉVISER |