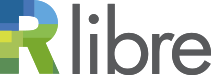
Répertoire de publications
de recherche en accès libre
de recherche en accès libre
Ben Nouma, Badreddine; Mitiche, Amar; Ouakrim, Youssef et Mezghani, Neila (2019). Pattern Classification by the Hotelling Statistic and Application to Knee Osteoarthritis Kinematic Signals. Machine Learning and Knowledge Extraction, 1 (3), 768–784. https://doi.org/10.3390/make1030045
Fichier(s) associé(s) à ce document :![]() |
PDF
- make-01-00045.pdf
Contenu du fichier : Version finale (non publiée) |
|
Catégorie de document : | Articles de revues |
---|---|
Évaluation par un comité de lecture : | Oui |
Étape de publication : | Publié |
Résumé : | The analysis of knee kinematic data, which come in the form of a small sample of discrete curves that describe repeated measurements of the temporal variation of each of the knee three fundamental angles of rotation during a subject walking cycle, can inform knee pathology classification because, in general, different pathologies have different kinematic data patterns. However, high data dimensionality and the scarcity of reference data, which characterize this type of application, challenge classification and make it prone to error, a problem Duda and Hart refer to as the curse of dimensionality. The purpose of this study is to investigate a sample-based classifier which evaluates data proximity by the two-sample Hotelling T2 statistic. This classifier uses the whole sample of an individual’s measurements for a better support to classification, and the Hotelling T2 hypothesis testing made applicable by dimensionality reduction. This method was able to discriminate between femero-rotulian (FR) and femero-tibial (FT) knee osteoarthritis kinematic data with an accuracy of 88.1% , outperforming significantly current state-of-the-art methods which addressed similar problems. Extended to the much harder three-class problem involving pathology categories FR and FT, as well as category FR-FT which represents the incidence of both diseases FR and FT in a same individual, the scheme was able to reach a performance that justifies its further use and investigation in this and other similar applications. |
Adresse de la version officielle : | https://www.mdpi.com/2504-4990/1/3/45 |
Déposant: | Mezghani, Neila |
Responsable : | Neila Mezghani |
Dépôt : | 10 oct. 2019 18:00 |
Dernière modification : | 10 oct. 2019 18:00 |
![]() |
RÉVISER |