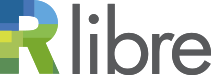
Répertoire de publications
de recherche en accès libre
de recherche en accès libre
Mabrouk, Rostom; Chikhaoui, Belkacem et Bentabet, Layachi (2018). Machine Learning Based Classification Using Clinical and DaTSCAN SPECT Imaging Features: A Study on Parkinson’s Disease and SWEDD. IEEE Transactions on Radiation and Plasma Medical Sciences, 3 (2), 170 - 177.
Fichier(s) associé(s) à ce document :
PDF
- IEEE-TRPMS_FINAL.pdf
Contenu du fichier : Manuscrit accepté (révisé après évaluation) Accès restreint |
|
Catégorie de document : | Articles de revues |
---|---|
Évaluation par un comité de lecture : | Oui |
Étape de publication : | Publié |
Résumé : | DatSCAN Single-photon emission computed tomography (SPECT) imaging is a reliable method to assess Dopaminergic Transporter in degenerative Parkinsonism. Scan without evidence of dopaminergic deficit (SWEDD) are subjects clinically diagnosed as Parkinson’s Disease (PD) patients although the SPECT imaging does not show any negro-striatal abnormality. In this paper, five models of machine learning were used to carry out binary classification (healthy control/PD) using clinical assessment and image-derived features applied thereafter on SWEDD group as a potential application of motor and non-motors features in understanding Parkinson disease characteristic in this group. The nested cross-validation was an essential component to select reliable models. A high accuracy was achieved for the five models (75.4% - 78.4% for motor features and 71% - 82.2% for non-motor features) in binary classification (HC vs PD). Cross all models applied on SWEDD group, 17.6% of patients were classified as PD motor disorder lookalikes, 27.4% were classified as having a beginning non-motor abnormality of PD and 3.9% were classified as having both motor and non-motor PD features. According to these facts, we demonstrate the suitability and usefulness of ML models to carry out binary classification of HC and PD SPECT data. However, the interpretability of SWEDD predicted condition must be carefully considered. |
Déposant: | Chikhaoui, Belkacem |
Responsable : | Belkacem Chikhaoui |
Dépôt : | 23 oct. 2018 14:21 |
Dernière modification : | 14 janv. 2020 15:51 |
![]() |
RÉVISER |