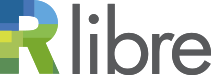
Répertoire de publications
de recherche en accès libre
de recherche en accès libre
Chikhaoui, Belkacem; Gouineau, Frank et Sotir, Martin (2018). A CNN Based Transfer Learning Model for Automatic Activity Recognition from Accelerometer Sensors. Dans Perner, Petra (dir.), Machine Learning and Data Mining in Pattern Recognition : 14th International Conference on Machine Learning and Data Mining MLDM 2018. New York, USA : Springer, coll. « Lecture Notes in Computer Science », vol. 10935. ISBN 978-3-319-96133-0 https://doi.org/10.1007/978-3-319-96133-0_23
Fichier(s) associé(s) à ce document :
PDF
- MLDM_2018_BChikhaoui_CRC.pdf
Contenu du fichier : Version finale (non publiée) Accès restreint |
|
Catégorie de document : | Communications dans des actes de congrès/colloques |
---|---|
Évaluation par un comité de lecture : | Oui |
Étape de publication : | Publié |
Résumé : | Accelerometers are become ubiquitous and available in several devices such as smartphones, smartwaches, fitness trackers, and wearable devices. Accelerometers are increasingly used to monitor human activities of daily living in different contexts such as monitoring activities of persons with cognitive deficits in smart homes, and monitoring physical and fitness activities. Activity recognition is the most important core component in monitoring applications. Activity recognition algorithms require substantial amount of labeled data to produce satisfactory results under diverse circumstances. Several methods have been proposed for activity recognition from accelerometer data. However, very little work has been done on identifying connections and relationships between existing labeled datasets to perform transfer learning for new datasets. In this paper, we investigate deep learning based transfer learning algorithm based on convolutional neural networks (CNNs) that takes advantage of learned representations of activities of daily living from one dataset to recognize these activities in different other datasets characterized by different features including sensor modality, sampling rate, activity duration and environment. We experimentally validated our proposed algorithm on several existing datasets and demonstrated its performance and suitability for activity recognition. |
Déposant: | Chikhaoui, Belkacem |
Responsable : | Belkacem Chikhaoui |
Dépôt : | 24 avr. 2018 20:04 |
Dernière modification : | 12 févr. 2025 13:53 |
![]() |
RÉVISER |